
Most college placement processes in India follow the same outdated cycle:
- Placement teams invite recruiters.
- Students submit resumes and sit for interviews.
- Recruiters filter through a large pool of students.
- A fraction of students get placed, while many remain unselected.
This system has major gaps:
- Students apply randomly, with little clarity on job fitment.
- Recruiters struggle to find candidates with the right skills.
- Placement teams lack data-driven insights to improve results year-over-year.
I worked on a project that aimed to fix these issues by leveraging AI, career analytics, and structured job-readiness programs to create a more effective placement system.
The Three-Part Framework for Smarter Placements
To reimagine placements, we focused on three key pillars:
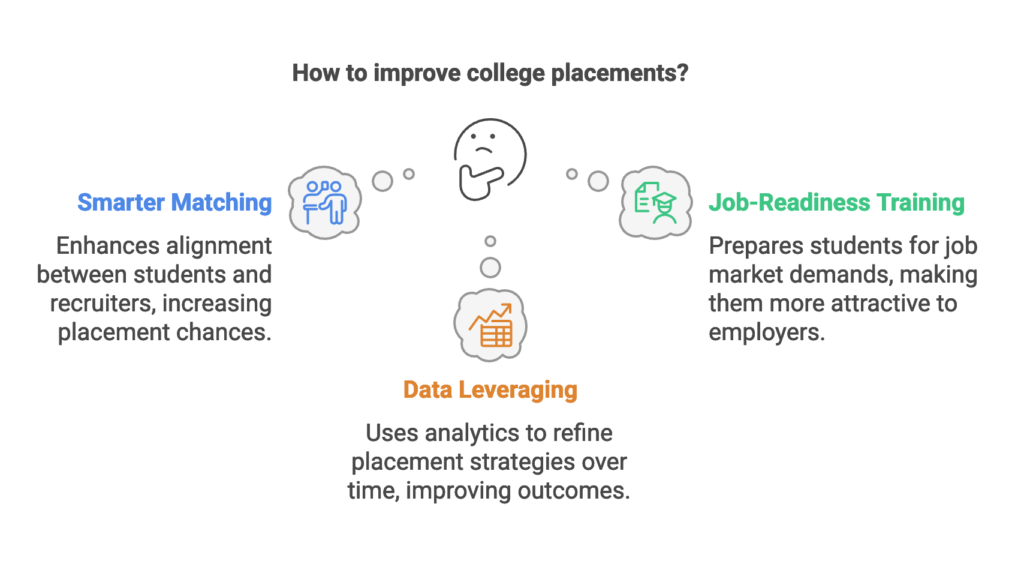
1. A Smarter Approach to Matching Students & Recruiters
The Problem:
- Recruiters needed job-ready students but had no way to pre-screen candidates efficiently.
- Students applied without understanding their career fit, leading to high rejection rates.
- Placement teams lacked a centralized system to manage recruiter-student interactions.
The Solution:
We developed an AI-driven framework that structured student-recruiter interactions more effectively:
Student Career Profiles: Instead of relying solely on resumes, a comprehensive student profile was built, including:
- Academic performance
- Internship history
- Skill-based assessments
- Career aspirations
Intelligent Job Matching: Recruiters could filter and shortlist students based on:
- Industry-specific skill sets
- Certifications and projects
- Performance in AI-based assessments
Placement Dashboard for Recruiters: Companies gained access to a centralized dashboard to:
- View pre-screened student profiles
- Schedule interviews
- Track hiring progress in real time
Outcome:
- Recruiters could now identify relevant candidates faster.
- Placement teams had better control over the job allocation process.
- Students received job recommendations aligned with their skills and interests.
2. Enhancing Student Job-Readiness with AI-Driven Training
The Problem:
Many students were not adequately prepared for recruiter interactions.
- Resumes lacked structure and failed ATS (Applicant Tracking System) scans.
- Students struggled in interviews due to poor communication skills.
- Job selection was random, with students applying for roles they weren’t fit for.
The Solution:
We designed a career prep module that focused on resume building, mock interviews, and skill-based assessments:
Resume Optimization Engine:
- AI flagged missing keywords based on recruiter job descriptions.
- Students received real-time feedback on structure, grammar, and industry relevance.
Mock Interview System:
- AI-based interview simulations evaluated responses for clarity, confidence, and technical accuracy.
- Students got instant feedback on areas of improvement.
Custom Skill-Building Tracks:
- Industry-focused training modules helped students strengthen in-demand skills like data analytics, digital marketing, and financial modeling.
- Personalized learning paths based on career aspirations and recruiter demand.
Outcome:
- Students entered placement season better prepared.
- Resume shortlisting rates increased for students who completed the AI-driven review.
- Recruiters spent less time screening unprepared candidates.
3. Leveraging Data to Improve Placements Over Time
The Problem:
Colleges traditionally do not track placement trends over multiple hiring cycles, leading to:
- Inconsistent recruiter engagement (some companies hire in bulk one year, then disappear).
- No visibility on hiring trends, making it hard to adapt to market demands.
- A lack of data-backed insights on why some students don’t get placed.
The Solution:
To solve this, we created a data-driven placement analytics system for universities to:
Track Recruiter Engagement Trends:
- Which companies returned consistently?
- What industries had high vs. low hiring activity?
Analyze Placement Success Rates:
- Which student profiles got placed fastest?
- What factors led to job rejections (skill gaps, interview performance, etc.)?
Forecast Future Hiring Demand:
- AI-based predictions on which industries will recruit heavily in the coming year.
- Recommendations for colleges to adjust training programs accordingly.
Outcome:
- Universities optimized placement strategies based on real data, not assumptions.
- Recruiters saw higher retention of hired students due to better role alignment.
- Placement teams could better prepare students for high-demand industries.
Key Takeaways for Colleges Looking to Improve Placements
This project highlighted a few critical lessons for universities:
- Move beyond resume filtering. AI-powered career profiles and job fitment models result in better recruiter-student matches.
- Make career training a structured process. Resume optimization, mock interviews, and skill training must be integrated into pre-placement prep.
- Use data to refine placement strategies each year. Universities need analytics on recruiter hiring patterns, student success rates, and skill gaps to optimize job placements.
The traditional mass-application approach is outdated.
Colleges need structured, data-driven placement systems to match students with the right employers, improve hiring rates, and build long-term recruiter relationships.
Final Thoughts: Why This Matters
Education is changing. The future of placements isn’t about volume—it’s about precision.
By implementing AI-driven matchmaking, structured job readiness training, and real-time analytics, we helped create a placement system built for long-term success.
Placements shouldn’t be a one-time event.
They should be an ongoing, data-driven process that evolves with industry needs.
This project was a step in that direction.